
Andrea Alamia
CerCo - Toulouse
COMPUTATIONAL NEUROSCIENCE
PREDICTIVE CODING, OSCILLATIONS and TRAVELLING WAVES
This project started with the work of Rufin VanRullen, presented at CCN 2017 (video presentation here). The original idea was that a simple 2-layers predictive coding model with physiologically plausible constraints can generate oscillations. Essentially, in a hierarchical predictive coding model, the higher layer tries to predict the activity of the lower layer, and the difference between the prediction and the actual activity (i.e. the prediction-error) is used to update the next prediction. By adding a physiological delay in the communication between layers, the prediction and its prediction-error will generate overshooting and compensatory mechanisms that ultimately give rise to oscillations.
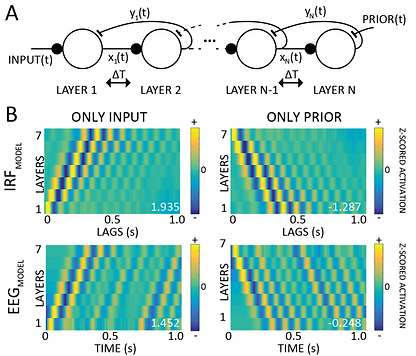
We extended this intuition to a multi-layer version, and we observed the rise of travelling waves (see figure). Remarkably, human-EEG recording from 2 dataset confirmed the presence of travelling waves. Moreover, as predicted by our model, waves' direction (either from lower to higher levels or viceversa) depends on whether participants are processing a sensory input (feedforward waves) or a resting with closed eyes (backward waves). For more details, check here our preprint.
Currently, in a series of 2 experiments we are investigating 1) the temporal dynamic of such waves and 2) its relation with visual awareness (by means of binocular rivalry).
EXPERIMENTAL PSYCHOLOGY
UNCONSCIOUS LEARNING
During my phd in Brussels I investigated unconscious processes under the supervision of Alexandre Zenon and Etienne Olivier. First we designed an experiment to robustly investigate the occurrence of unconscious learning during a perceptual decision making task (here the paper). Once we reliably assessed that the learning was actually unconscious, we exploited this paradigm to investigate 1) the role between visual attention and unconscious processes (here) and 2) its physiological correlate in motor cortex (and here).
Currently, with Rufin VanRullen, we are investigating which neural networks architecture matches human learning in an Artificial Grammar Learning (AGL) task. AGL tasks are widely used to investigate both implicit learning and language acqusition. In this study, we compared human learning with FEEDFORWARD and RECURRENT networks across three layers of the Chomsky's Hierarchy (Regular, Context-free and Context-specific grammars). Preliminary results of this work have been presented (poster) in Barcellona at the "HBP conference 2018 : Understanding Consciousness".